Now that we’re well into the age of AI and machine learning, the ability to foresee business outcomes and use data to inform decisions is not just an advantage — it’s a necessity.
Predictive modeling enables more accurate forecasting, a deeper understanding of customer behaviors, and stronger marketing performance. From uncovering the most promising accounts to optimizing resources and enhancing marketing ROI, predictive modeling opens doors to unprecedented insights and opportunities.
Join us as we explore predictive modeling, including its applications, methodologies, and challenges.
What is Predictive Modeling in Marketing?
Predictive modeling is a powerful technique used in B2B marketing to forecast future outcomes and make data-driven decisions. This approach helps businesses gain valuable insights into customer behavior, market trends, and campaign performance by analyzing historical data and identifying patterns.
Using this approach, marketers can go beyond traditional methods of analysis and use advanced algorithms to allocate resources effectively and improve marketing ROI.
Example of a Predictive Model
Acme Corp., a fictional SaaS company, wants to identify the most promising accounts for their new product launch. Looking at historical data, its predictive model considers factors such as:
- Company size
- Industry
- Past purchase behavior
- Marketing engagement
Based on this analysis, the predictive model assigns a lead score to each potential customer, indicating their likelihood of converting into a paying customer. The sales team can then prioritize their efforts by focusing on leads with higher scores, increasing their chances of closing deals and maximizing revenue.
How Does Predictive Modeling Work?
Building a predictive model involves a series of steps that help marketers make informed decisions. Here’s a breakdown of the key actions for developing a predictive model.
- Identify objectives. Determine what specific marketing challenge or goal you want to address with the predictive model. Whether it’s improving lead conversion rates, optimizing campaign performance, or facilitating customer segmentation, a well-defined objective sets the foundation for the entire modeling process.
- Gather and prepare data. Success of a predictive model relies on the quality and relevance of the data used. Collect this from various sources such as customer databases, CRM systems, and marketing automation platforms. Clean and preprocess this data to remove inconsistencies and errors.
- Select variables. Identify what factors are most likely to influence outcomes. These include firmographic data, purchase history, and industry-specific metrics. Carefully select those that have the highest predictive power and eliminate any that may introduce noise or bias into the model.
- Build and train the model. Choose an appropriate algorithm and train the model using historical data. The algorithm learns from patterns and relationships within the data to make predictions. Regularly adjust parameters and evaluate performance until the model achieves the desired level of accuracy.
- Validate the model. Bring in data that was not used during the training phase to test the model’s predictions. This ensures that the model can generalize well to unseen data and provides reliable predictions. Evaluate the model’s accuracy, precision, and recall to assess its effectiveness.
- Deploy and monitor. Integrate the predictive model into your existing systems and workflows. Use it to generate real-time predictions and recommendations for lead generation, sales targeting, or customer segmentation. Continuously monitor performance and update as needed to adapt to changing market dynamics and customer behavior.
Types of Predictive Models
Predictive modeling in marketing offers various powerful techniques that help organizations achieve better outcomes. Here are five types to consider.
Linear Regression
Linear regression establishes a linear relationship between the dependent variable and independent variables, making it useful for predicting outcomes based on factors like:
- Advertising spend
- Website traffic
- Customer firmographics
A software company may use linear regression predictive modeling to forecast sales revenue for the upcoming quarter. By analyzing historical data like marketing spend, number of sales calls, and target audience size, this model can be built to identify the relationship between these variables and sales revenue. This insight empowers the organization to make informed decisions about resource allocation and marketing budget planning.
The linear regression model will look something like this:
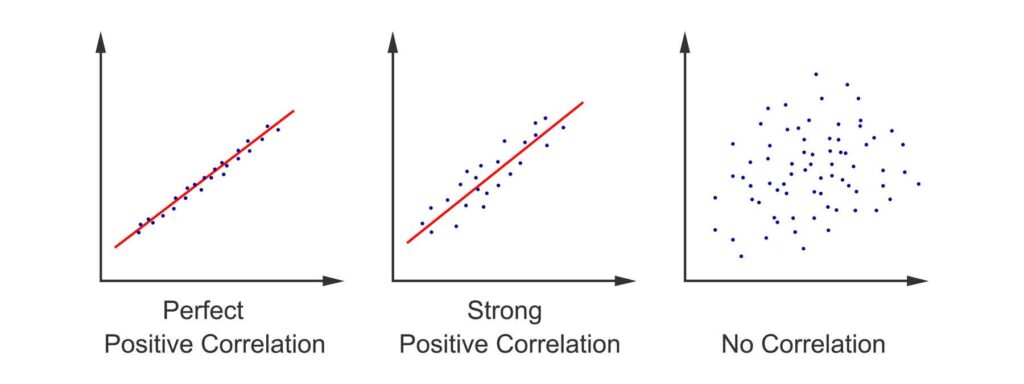
Decision Trees
Decision trees are intuitive models that use a tree-like structure to make predictions. They provide transparency and insights to facilitate complex decision-making. For the Gen Xers and Millennials, this model may remind you of the quizzes offered in late 90s/early 2000s teen magazines like, “Which Boy-Band Heartthrob is Your Soulmate?”
While you and Joey McIntyre may not have worked out, decision trees are much more accurate when it comes to making marketing predictions. For instance, a company may use a decision tree to predict the likelihood of a lead converting into a customer by considering factors such as industry, company size, and marketing engagement.
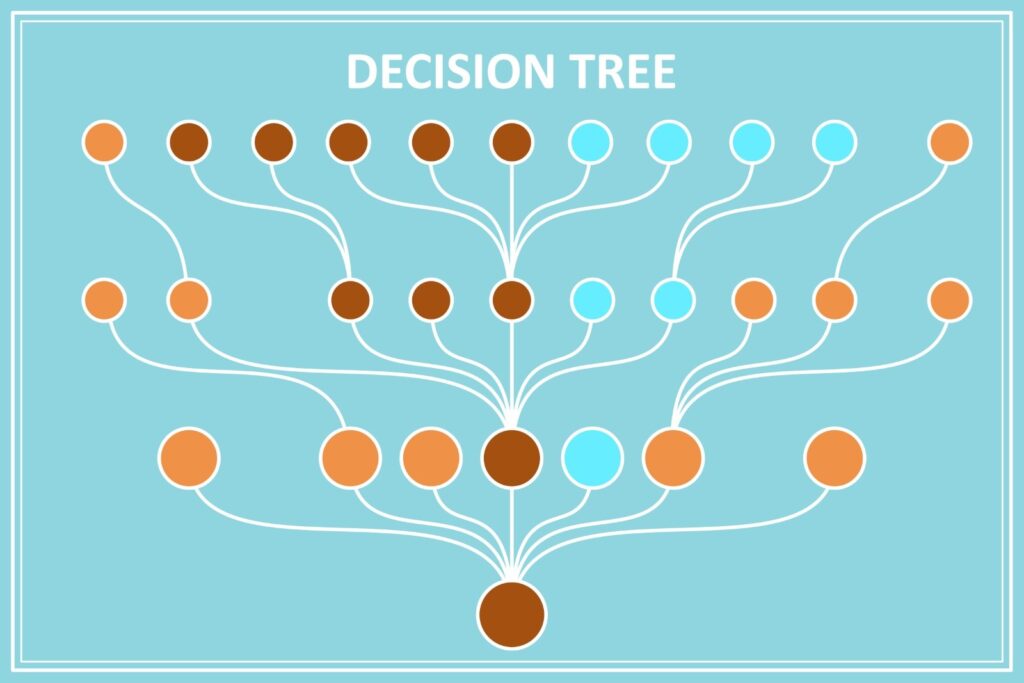
Random Forests
Random forests combine multiple decision trees to make predictions. They’re particularly effective in handling large and diverse datasets, making them suitable for organizations dealing with complex inputs. Random forests can be used to predict customer churn by considering factors like:
- Contract renewal history
- Customer support interactions
- Customer behavior
Having insight into customers who may be considering other solutions enables you to provide appropriate outreach and messaging.
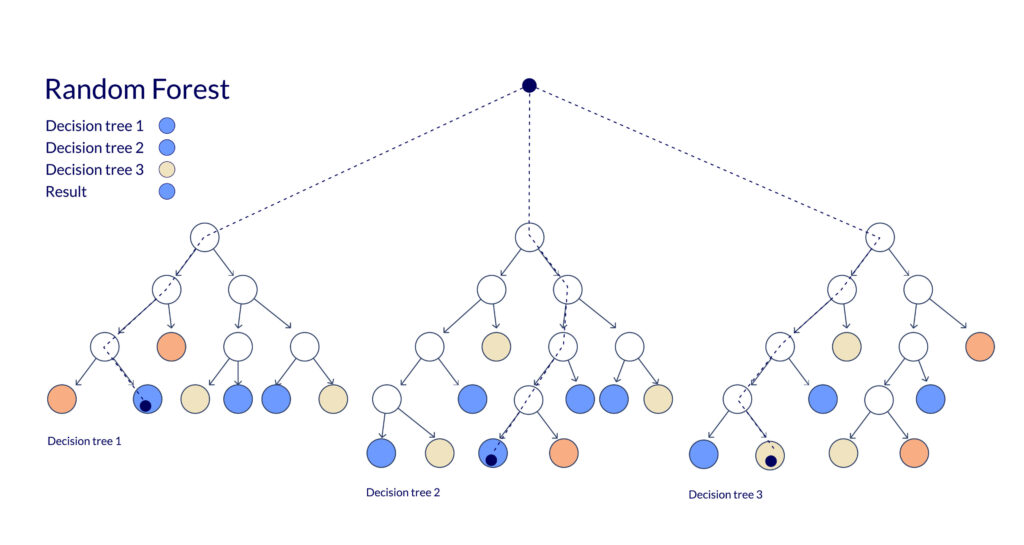
Neural Networks
Inspired by the neural structure of the human brain, neural networks are powerful models capable of learning complex patterns and relationships in data.
Results will look something like this (if you’re prone to migraines, we recommend skipping ahead):
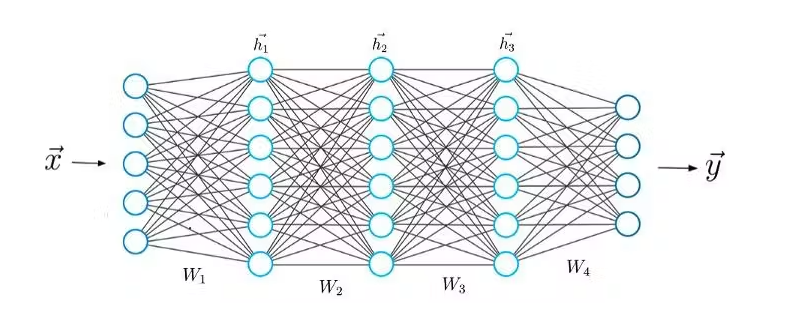
Fortunately, integrating this predictive model means you don’t have to look at the mess; you just get to take advantage of the valuable insights extracted from it.
Support Vector Machines (SVM)
SVM is an effective technique for organizations with sophisticated datasets and a multitude of variables. We could spend pages and hours explaining the intricacies — but put simply, it cross-references all data points to find the optimal boundary for separating and classifying the information. Organizations can use this model to predict whether a lead will convert into a customer based on factors like:
- Industry
- Company size
- Past purchase behavior
- Other technologies used
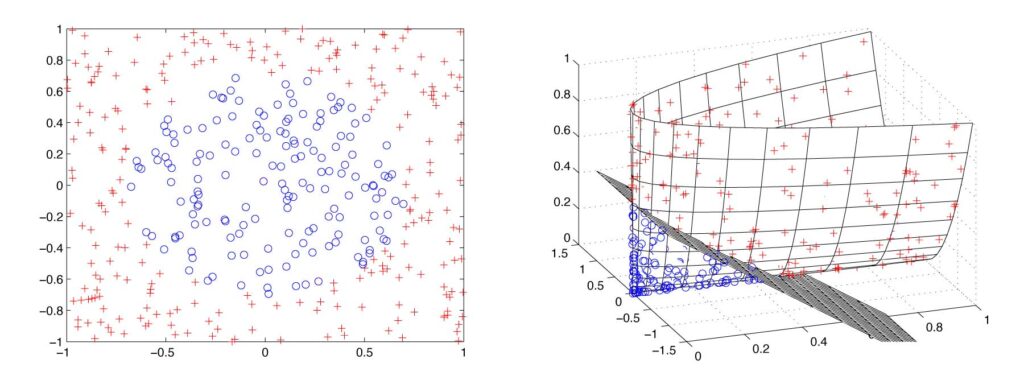
Challenges of Predictive Modeling
As is the case with many B2B tools, predictive modeling comes with its own set of obstacles.
Data Quality and Availability: Incomplete or inaccurate data can lead to unreliable predictions.
How 6sense helps: We use a vast data network, which collects and analyzes data from various sources to provide comprehensive insights.
Complexity and Interpretability: When results from predictive models are complex or difficult to understand, sales and marketing have difficulty acting upon the insights.
How 6sense helps: We simplify the process by providing user-friendly dashboards and visualizations that make it easy for teams to understand and use the predictions.
Scalability and Integration: Predictive modeling has various use cases for organizations. It can be daunting to both scale across multiple departments and teams and integrate with existing systems.
How 6sense helps: Our scalable solution seamlessly integrates with numerous CRM and marketing automation platforms, ensuring organizations can use predictive insights across their entire sales and marketing ecosystem — enabling consistent and data-driven decision making.
Maintenance and Adaptability: Models require continuous updating and adapting to reflect changing market dynamics and customer behavior.
How 6sense helps: Our advanced AI algorithms automatically learn and adapt to new data patterns, ensuring that models remain accurate and relevant over time.
Empowering the Future of Business
6sense Predictive Analytics is a game-changer for sales and marketing teams. The platform’s effectiveness lies in its ability to accurately predict customer behavior, identify high-value prospects, and optimize marketing campaigns.